What’s Media Mix Modeling? [Marketer’s Guide with Examples]
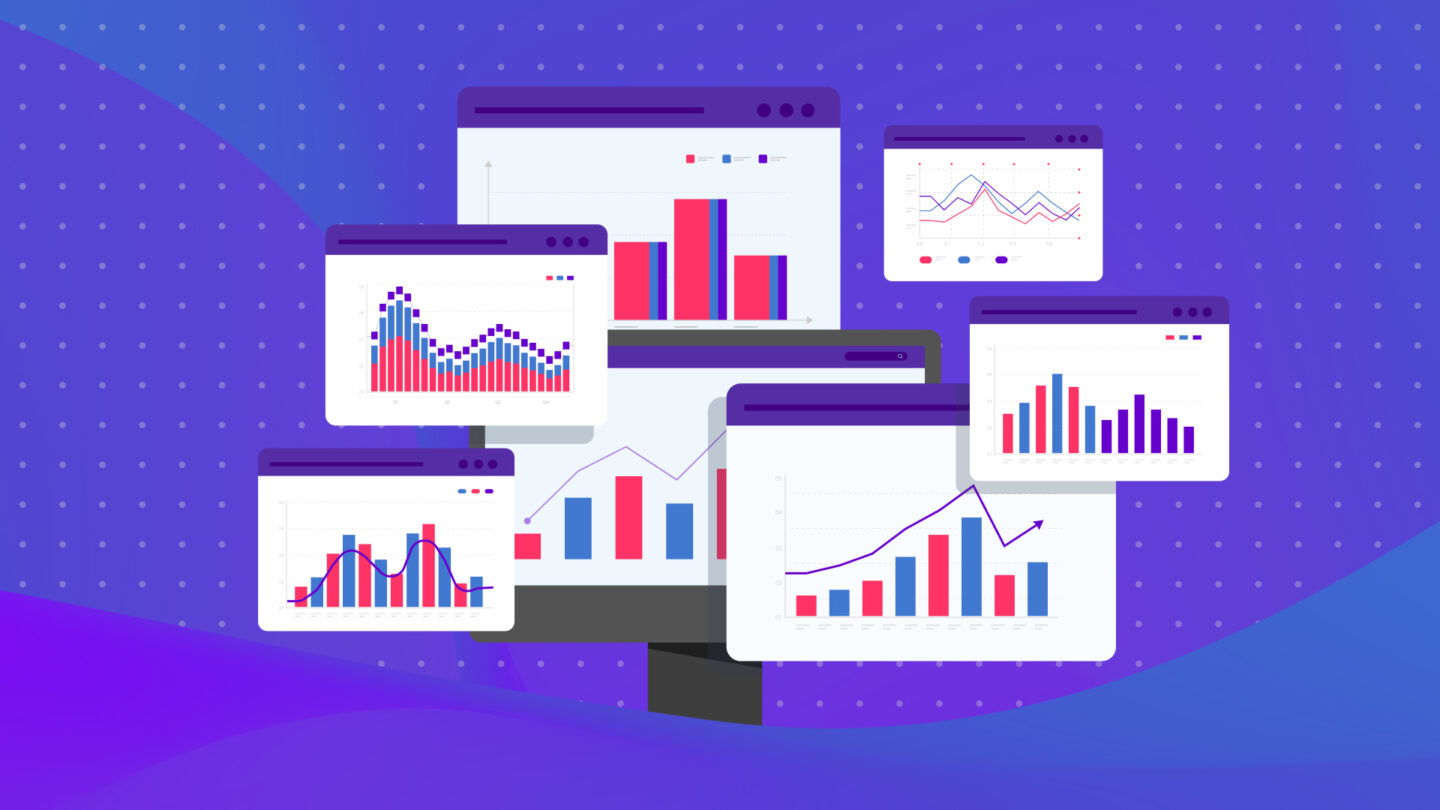
Have you ever felt in the dark when it comes to understanding the real impact your marketing dollars are having across multiple channels?
Determining where and how conversions are occurring is crucial in optimizing your budget to drive the most impact with your marketing budget. Media mix modeling (MMM) is an analytical approach used to gauge the effectiveness of various marketing channels in driving sales and conversions. This method allows us to decipher the true influence of advertising spend across diverse platforms by accounting for a myriad of factors, both within their control (like media channel spend, promotional strategies) and outside their control (such as economic conditions, competitor actions, and seasonal influences).
One of the key strengths of media mix modeling is its ability to incorporate long-term brand building effects alongside immediate sales impacts, offering a comprehensive view of marketing effectiveness. It helps in identifying which channels are most efficient, how different channels influence each other, and how external factors affect marketing performance.
Media mix modeling is a powerful tool for marketers seeking to optimize their marketing investments. By providing a holistic view of how various factors contribute to sales and conversions, MMM enables data-driven decisions that enhance marketing efficiency and business growth.
In this article, we explore how media mix modeling works, and how businesses can use analytics to drive smarter ad spend decisions.
Media mix modeling (MMM) is a type of analysis that measures the impact of media buys across multiple channels, showing the role various elements play in achieving a desired outcome—often a conversion or revenue KPI. With this information, marketing stakeholders are able to make specific adjustments to campaign spend to improve their progress toward reaching a given goal.
Media mix modeling can be used to address common brand marketing questions and pain points, including:
“Media mix modeling is a top-down , privacy resilient approach that evaluates how historical media activity, promotions, pricing, seasonality, and uncontrollable factors—such as economic activity—impact key business outcomes such as sales revenue. MMM is a scientific approach to attribution in the sense that it applies statistical methods to analyze and interpret marketing data, providing a systematic understanding of how different marketing channels contribute to overall business goals in the broader context of the market. The quality of insights derived from MMM heavily depends on the quality and granularity of the data used.”
— Annica Nesty, Group Director of Marketing Science at Tinuiti
MMM leverages aggregate data, and can measure both online (digital) and offline (traditional) advertising channel performance, including (but not limited to): paid media channels such as social media channels, traditional print advertising, linear TV advertising, and other performance marketing efforts, organic media, operational factors like promotions, external factors like seasonality, economic conditions, outcome KPIs such as sales revenue, new customers, and conversions.
The MMM framework is a type of statistical analysis that uses statistical methods and econometric models such as a regression analysis. This econometric model helps analysts determine the strength of relationships between a single dependent variable and an array of independent variables.
Media mix modeling analysis measures the impact of your media spend today, and is also helpful in predicting the future outcome of your marketing investments on a given variable.
Example:
Let’s assume a scenario where our target metric, or dependent variable, is revenue, a critical indicator of business success. We aim to dissect the influence of various marketing initiatives on this revenue. These initiatives, our independent variables, encompass a diverse array of digital advertising campaigns, including those run on TikTok, Instagram, Snapchat, as well as broader Display and Streaming platforms.
The number of independent variables under scrutiny does not dilute our core objective. The mission is to measure the relationship between the marketing endeavors and the revenue they generate. This involves not only identifying the direct contributions of each campaign to revenue but also understanding the nuanced interplay between them by observing how changing aspects of those independent variables impacts the chosen business outcome
When using MMM to assess campaign success, marketers should leverage statistical methods and econometric models to get the most accurate picture possible. Data quality is essential in achieving an accurate media mix analysis, so take any needed time to clean your data before using it in your analysis.
Key elements an MMM equation can measure include:
Like media mix modeling, attribution modeling also studies the efficiency of marketing strategies — but there are important differences.
Attribution modeling is a general term that refers to tracking engagement to better understand how specific tactics drive action at the user level. This modeling works well for analyzing specific customer touchpoints, focusing on elements like how a consumer converted, which creative on which channel led to that conversion, and what the expected ROI could be if more ad budget were shifted to that channel.
Media mix modeling takes a higher-level, more comprehensive picture. This modeling isn’t designed to measure user-level engagement like impressions and clicks, rather its primary function is measuring the impact of an entire touchpoint on specific marketing objectives.
Data-driven attribution modeling and MMM each have their own set of strengths. It’s not a matter of one being better than the other, rather one being better-suited to different types of marketing analysis.
For example:
“Attribution modeling is based on a bottom-up approach while media mix modeling takes a top-down approach. Media mix modeling provides a long-term view of the marketing ROI of media activity, while attribution modeling evaluates individual-level activity to provide a short term view of marketing ROI.”
— Annica Nesty, Group Director of Marketing Science at Tinuiti
In the post-cookie and post-IDFA landscape, where privacy concerns and regulatory changes limit access to individual user-level data, media mix modeling has become a pivotal analytical tool. MMM’s emphasis on overall marketing spend allocation and its proficiency in establishing cause-and-effect models, address the challenges posed by the diminishing availability of explicit conversion information, providing marketers with a privacy-respecting and insightful approach to navigate the evolving digital advertising ecosystem.
With the right media mix model, a business can measure their past marketing performance to improve future ROI by optimizing the allocation of the media budget by channel and/or tactic, including: traditional and digital media channels, promotions, pricing, competitor spend, economic conditions, weather, and more.
Example:
An international ecommerce brand wanted to forecast their second-half of the year and create an optimal media mix to make their marketing dollars work smarter. A combination of client data, marketing data, and machine learning were required to create a powerful, custom media mix model.
To build the model, the business used 2+ years of digital marketing and revenue data, analyzing it by market, tactic, and day. The data was then used to create model to assess future spend showing how changes in investment across channels could impact revenue and sales.
The full digital media mix model gave the ecommerce brand a detailed analysis of where to optimize their spend across all digital marketing channels.
One recommendation was to shift dollars away from social—which historically had been at or near 30%—to paid search. This recommendation came with another layer of insight: The brand realized they were overinvesting in awareness campaigns, and needed to invest more heavily in capturing current demand during the 2nd half of the year.
Results: Working with a robust media mix model, the brand was able to break down how much media spend was needed by each channel in order to achieve the 30% YoY revenue goal they targeted.
MMM helps you accurately connect all the dots, leveraging (ideally) a wealth of provided data, to understand how disparate aspects of marketing campaigns work together in helping you reach your business goals.
The benefits of MMM are multifaceted, offering marketers a strategic edge in navigating the intricacies of their advertising efforts. Let’s dive into each benefit in detail…
Omnichannel Campaigns: MMM excels in providing insights for omnichannel campaigns, allowing marketers to understand and optimize the impact of their initiatives across various channels. This capability is crucial in today’s interconnected digital landscape, where consumers engage with brands through diverse platforms.
Improved Oversight Over Media Spend Impact: MMM provides a comprehensive view of the impact of media spend, enabling marketers to assess the effectiveness of their investments. This improved oversight ensures a clearer understanding of how each component of the media mix contributes to overall campaign success.
Media Spend Optimization: With MMM, marketers can optimize their media spend by identifying the most impactful channels and touchpoints. This data-driven approach allows for strategic adjustments in budget allocation, ensuring that resources are directed towards the avenues that yield the highest return on ad spend.
Effective Targeting of Audiences: MMM’s analysis helps in refining audience targeting strategies. By understanding which elements of the marketing mix resonate most with specific demographics, marketers can tailor their campaigns to effectively reach and engage their target audience segments.
Forecasting with Certainty: One of MMM’s strengths lies in its ability to forecast results with a high degree of certainty. This forecasting capability empowers marketers to make informed decisions based on predictive analytics, aiding in long-term planning and goal setting.
Reduced Reliance on Personally Identifiable Information (PII): MMM minimizes the reliance on personally identifiable information for analysis. This is especially crucial in an era where privacy concerns are more important than ever.
Media mix modeling is a comprehensive and powerful tool, offering a range of benefits that contribute to a more effective, data-driven, and privacy-conscious approach to marketing strategy and decision-making. While there are many benefits to MMM, there are challenges as well. Let’s look into common challenges of MMM in our next section.
MMM grows increasingly complex as the media landscape becomes more fragmented, and the customer journey more personalized. Whereas in the past, advertisers may have wanted to measure something as simple as the impact of a print ad in a Cleveland newspaper, today’s consumers are exposed to brands in a wide variety of locations and formats, from a subway transit poster to a Sponsored post on Instagram.
Working with high-quality data is important in any measurement initiative, but for MMM to work effectively, it also needs a lot of data to build a reliable model. For example, if you wanted your model to consider the performance impact of seasonality, it would ideally need at least three full seasons (three years) of data to consider in its analysis.
This makes media mix modeling a ‘long game’ initiative with infrequent reporting by its nature. Brands and advertisers who are more accustomed to daily or weekly updates may struggle with ‘waiting out’ the analysis.
Because it’s not designed to make considerations based on user-level data, instead providing aggregate insights, media mix modeling offers limited insights on brand impact, personalized targeting, and customer experience. However, advanced models are available that can provide highly granular insights, but traditional MMM provides aggregate insights.
Media mix modeling, like many other analytics solutions, has also become a marketing buzzword that has generated its fair share of misconceptions.
Here are a few of the most common misconceptions around media mix modeling.
With large datasets and statistical analysis involved in media mix modeling, the methods behind the technique have been critiqued for their obscurity. If there is no perceived transparency in the process, how does a brand know if its media mix model is really accurate?
Any organization specializing in media mix modeling should provide a transparent approach, with deliverables such as outlines, milestones, and performance reports. Additionally, you may want to consider partnering with an agency that truly understands how media mix modeling aligns with your needs and expectations. Every business is unique and each media mix model is based on multiple factors.
Today, results are often measured by the timeliness of their delivery, with the current digital marketplace allowing for almost instantaneous real-time data. Media mix models do actually provide compelling real-time marketing insights, perfect for evaluating new campaigns, new competitors, and assessing pricing actions or changes in promotional strategies.
A powerful partner in media mix modeling will provide sophisticated tools and real-time approaches to satisfy your business performance assessments. Your partner should also be able to provide forecasting, simulation, or AI- and machine-learning-integrated models to suggest future movements.
Though media mix strategies do integrate and consider offline channels in their approaches, media mix modeling also considers all digital channels — including display, email, paid search, social, and more. Remember—it’s considering your media mix. If that includes ten different channels and you provide enough high-quality data for each, they will all be considered in your marketing mix analysis.
In fact, as customers have become more intertwined with digital channels, media marketing models have adapted to go even deeper into the analyses provided by those channels’ respective insights to support better budgeting choices and customer segmentation reports.
In an ever-evolving digital landscape, MMM’s adaptability to the post-cookie/post-IDFA world positions it as an essential tool for marketers. As businesses seek to connect the dots, leverage data, and make strategic decisions, MMM is a crucial ally in the dynamic realm of mixed media advertising.
“At Tinuiti, we leverage measurement best practices such as MMM and incrementality to understand media effectiveness, predict future outcomes, create deeper insights, analyzing what-if scenarios to provide recommendations that optimize media performance. This helps brands understand what channels they should be investing in, how they should shift budgets (media mix), creating a high-level view of what channels are driving overall sales and ROI. Our goal here is to deliver growth for our clients by maximizing the return on investment through best in class measurement”
— Annica Nesty, Group Director of Marketing Science at Tinuiti
At Tinuiti, we know, embrace, and utilize MMM. Our Rapid Media Mix Modeling sets a new standard in the market with its exceptional speed, precision, and transparency.
Our proprietary measurement technology, Bliss Point by Tinuiti, allows us to measure what marketers have previously struggled to measure – the optimal level of investment to maximize impact and efficiency. But this measurement is not just to go back and validate that we’ve done the right things. This measurement is real-time informing what needs to happen next.
Curious about how we can tailor strategies to hit your unique marketing bliss point, including Rapid Media Mix Modeling? We’re eager to chat. Contact us today for details.